Facial recognition technology has gained significant attention in recent years for its potential applications in various fields, including law enforcement, security, and convenience. However, concerns about the fairness and bias associated with this technology have also emerged. This blog explores the question: Is facial recognition biased? We will delve into the factors contributing to bias in facial recognition systems and the implications of these biases on individuals and society.
Understanding Facial Recognition Technology(FRT)
Facial recognition technology uses algorithms to identify and verify individuals based on their facial features. It has become increasingly popular in applications such as unlocking smartphones, access control systems, and surveillance cameras. However, the accuracy and reliability of these systems have been called into question due to their susceptibility to bias. The large datasets used to train facial recognition models may have imbalances in terms of racial and ethnic diversity, may have very limited data on minorities. The way facial features are extracted and represented can impact bias. If the algorithm emphasizes certain features more than others based on the training data, it may perform better on faces that match those features and worse on faces that deviate from them.
Facial recognition systems used today tend to show higher accuracy rate at white, young and male faces
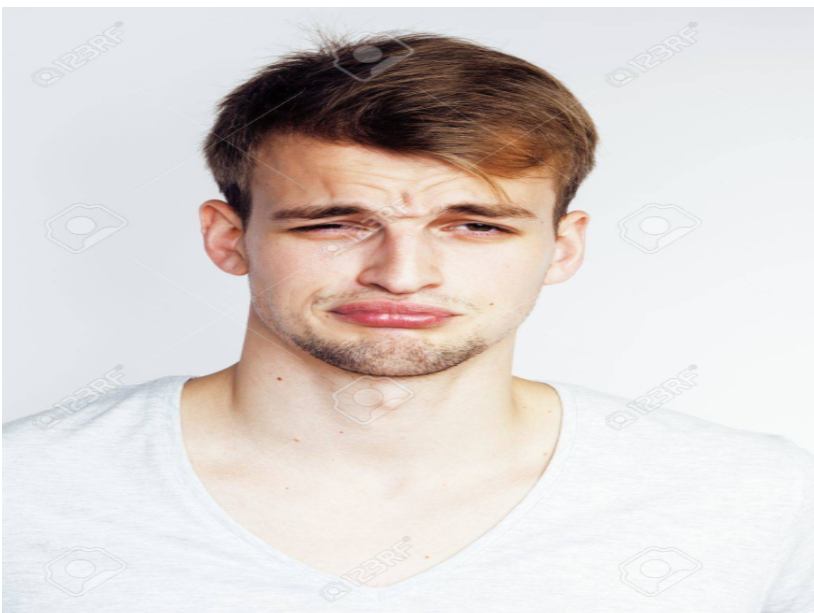
And the accuracy rates fall to the lowest when dealing with coloured, old and female faces
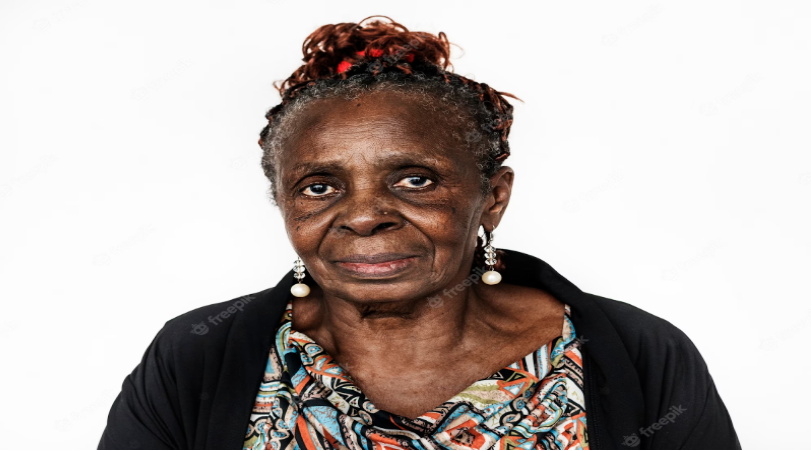
These things happen because of the different forms of biases that find their way into various datasets & there are also a few technical reasons that contribute to this. But the most alarming thing about this situation is the 35% difference in accuracy that is observed across races, genders and age brackets. This can have a reinforcing effect on the pre-existing racism in most societies. The already vulnerable groups like blacks and hispanics in America may find themselves on the wrong side of the law more often because of such biases ingrained in these technologies. Errors in facial recognition algorithms can disproportionately affect certain groups. For example, if the algorithm has a higher rate of false positives or false negatives for a particular racial group, it can result in bias against that group.
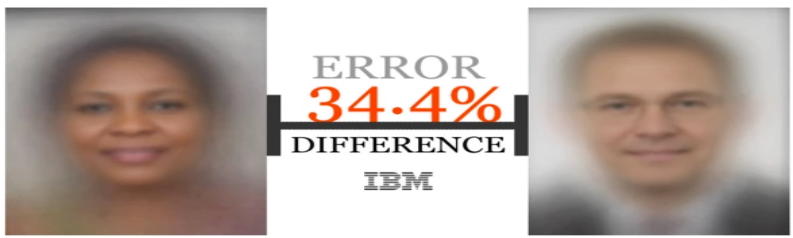
Factors Contributing to Bias:
Data Bias: Facial recognition algorithms rely on vast datasets to learn and identify faces. These datasets are often collected from the internet, which can introduce bias as they may not accurately represent the diversity of the population. If the training data is primarily composed of certain demographics, such as white males, the system may perform poorly when recognizing faces from underrepresented groups.
Algorithmic Bias: The design of facial recognition algorithms can also contribute to bias. If developers do not consider the potential for bias during the algorithm’s creation, it may inadvertently discriminate against certain racial, gender, or age groups. For instance, some algorithms have been found to have higher error rates when identifying people with darker skin tones or non-binary gender expressions.
Human Bias: Human biases can enter facial recognition technology at multiple points in the development process, from data collection to algorithm design and testing.
Biased human judgments can affect the quality of training data and lead to biased outcomes. Few of such biases include:
1. Racial Bias: Several studies have shown that facial recognition algorithms tend to perform less accurately on individuals with darker skin tones. This racial bias can lead to misidentifications, unfairly targeting minority communities.
2. Gender Bias: Facial recognition systems have been known to misclassify individuals based on their gender, particularly transgender and non-binary individuals. This can result in discomfort and discrimination.
3. Age Bias: Accuracy can decrease significantly when facial recognition is applied to older adults, potentially impacting the security and convenience of aging populations.
4. Dataset Bias: One of the primary sources of bias in facial recognition lies in the data used to train these algorithms. If the dataset predominantly consists of certain demographics, the technology becomes skewed toward those groups.
5. Privacy Concerns: Beyond bias, facial recognition raises substantial privacy concerns. The ability to track and identify individuals without their consent can lead to invasive surveillance practices.
6. Civil Rights and Discrimination: Biased facial recognition systems can exacerbate existing societal biases and inequalities. It can disproportionately impact minority groups and result in discriminatory practices in law enforcement and other sectors.
7. Inaccuracy in Law Enforcement: The use of facial recognition in law enforcement has garnered significant attention. If the technology is biased, it can lead to wrongful arrests, racial profiling, and miscarriages of justice.
8. Erosion of Trust: The existence of biased facial recognition systems erodes trust in technology and institutions. It can also lead to public backlash and calls for greater regulation.
To mitigate bias in facial recognition technology, several steps can be taken:
Diverse and Representative Data: Ensuring that training data is diverse and representative of all demographic groups is crucial. Developers should be diligent in collecting and curating data to reduce bias.
Transparent Algorithms: Developers should make their algorithms transparent and subject them to third-party audits to identify and rectify any biases. Transparency can also help build trust with users and the public.
Regulation and Oversight: Governments and regulatory bodies can implement laws and guidelines to govern the use of facial recognition technology. These regulations should address issues related to bias, privacy, and civil rights.
Facial recognition technology has the potential to revolutionize various industries, but its bias issues must be addressed to ensure fair and equitable use. The question, “Is facial recognition biased?” is not a simple one to answer, as bias can manifest in various ways throughout the technology’s development and application. To move forward, stakeholders must collaborate to develop and implement measures that reduce bias and safeguard individuals’ rights and privacy.
Read more at
Buolamwini, J., & Gebru, T. (2018). Gender Shades: Intersectional Accuracy Disparities in Commercial Gender Classification. Proceedings of Machine Learning Research, 81, 1-15.
Smith, A. N., & Jain, A. K. (2021). Biases in Face Recognition: A Review. arXiv preprint arXiv:2105.13406.
Garvie, C., Bedoya, A., & Frankle, J. (2016). The perpetual line-up: Unregulated police face recognition in America. Georgetown Law, Center on Privacy & Technology.
ACLU. (n.d.). The Fight Against Face Surveillance. Retrieved from https://www.aclu.org/issues/privacy-technology/surveillance-technologies/fight-against-face-surveillance